Successful dissertation defense of Mohammad Zenouzzadeh
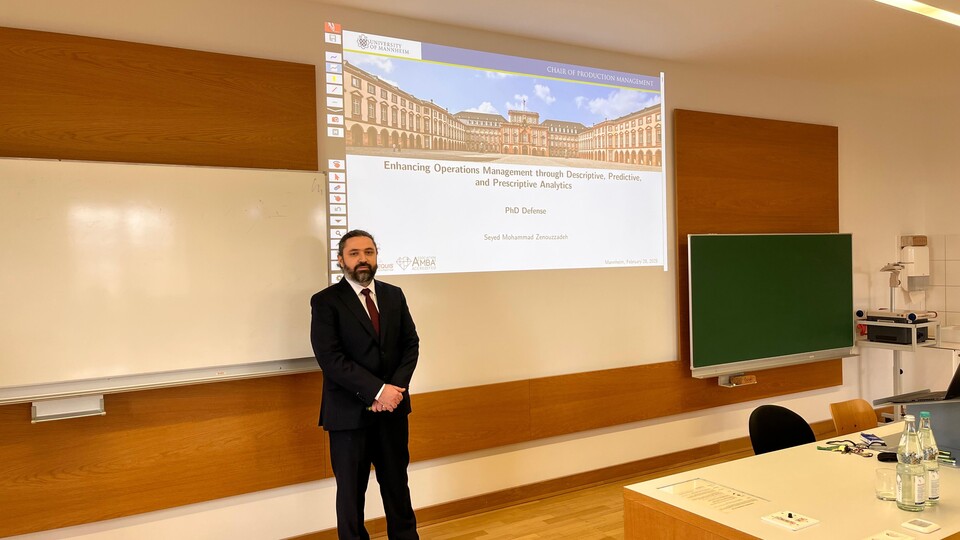
On Friday, Febraury 28, 2025, Mohammad Zenouzzadeh successfully defended his dissertation entitled: „Enhancing Operations Management through Descriptive, Predictive, and Prescriptive Analytics“.
The essays' titles are: „Pay-Per-Second vs. Listening to Ads: Analyzing Advertisement Effects on Customer Patience in Call Centers“, „A Machine Learning Approach for the Analysis of Time-Dependent Queues: ML4TDQ“, „Shift Scheduling with Transfers between Interdependent Departments“.
Congratulations, Mohammad!
Abstracts:
Pay-Per-Second vs. Listening to Ads: Analyzing Advertisement Effects on Customer Patience in Call Centers
We analyze empirical data from a French connecting call center operating under two business models: pay-per-second calls and calls with advertisements during waiting time. We begin with data cleaning and basic statistics to provide an overview of the datasets. Then, we employ existing methods to analyze censored customer patience, revealing a significant difference in customer behavior when advertisements are present. We find that the initiation of advertisements triggers a wave of call abandonment, suggesting customer disinterest in ads and possibly irrelevant content. Furthermore, a secondary abandonment wave occurs post-advertisement, likely due to customers expecting immediate service after the end of advertisement. Notably, in the advertisement dataset, a counter-intuitive negative relationship between average waiting time and call abandonment is observed, which we attribute to the sunk cost phenomenon. Customers perceive listening to advertisements as an investment, prompting them to remain on the line for service. We present the first empirical study on the effect of advertisements on customer patience. These insights benefit call center managers and serve as a foundation for developing queueing models to capture this effect.
A Machine Learning Approach for the Analysis of Time-Dependent Queues: ML4TDQ
Most real world queueing systems operate in a time-dependent environment whether due to seasonality (e.g., time of day or month of the year) or long-term changes to the environment caused by changes in the system configuration or parameters. Analysis of these time-dependent systems can be performed through the analysis of the transient behavior of queues with timeinvariant parameters. We propose an algorithm to combine transient analysis models iteratively to estimate the behavior of time-dependent queues. For the transient analysis of queues, we propose two methods. The first method uses machine learning to estimate the transient performance measures and the second method combines machine learning with domain knowledge in a two-step framework to be more explainable and remedy some well-known shortcomings of machine learning methods, e.g., being uninterpretable and overfitting. Lastly, through extensive numerical experiments, we show the effectiveness of the methods proposed in this work in the analysis of time-dependent queues.
Shift Scheduling with Transfers between Interdependent Departments
Many multi-stage manufacturing, logistics, or service systems, for example, distribution centers, require differently trained workers per stage. Workforce scheduling for such systems has to consider the heterogeneity in workforce and the interdependency of stages or departments due to material or customer flow. To increase efficiency, it could be favorable to transfer workers from one stage to another during their shifts, even if the transfer time leads to capacity losses.
To analyze the trade-off between loss of effective capacity and additional flexibility provided because of the inter-department transfers, we formulate the daily shift scheduling problem as a mixed-integer programming (MIP) model, considering heterogeneous workforce, interdependent stages, and the possibility to transfer workers between stages within the shifts. These intra-shift transfers lead to a reduction in effective processing time. Under the constraint that the timedependent demand has to be fulfilled by the end of the planning horizon, the objective is to minimize the cost for contingent workers. Moreover, a column generation approach is developed to efficiently solve the problem, especially for large-sized instances.
For a realistic instance from a distribution center, we demonstrate how transfers can result in cost savings. In addition, we conduct numerical experiments to demonstrate the impact of the level of transfer flexibility on the structure of the optimal solution and on the relevant costs. Computational experiments indicate that the algorithm can provide good solutions within reasonable times.